Responsible AI Deployment: The Essential Role of Data Management
Authored by EncompaaS - Mar 5, 2024
Share
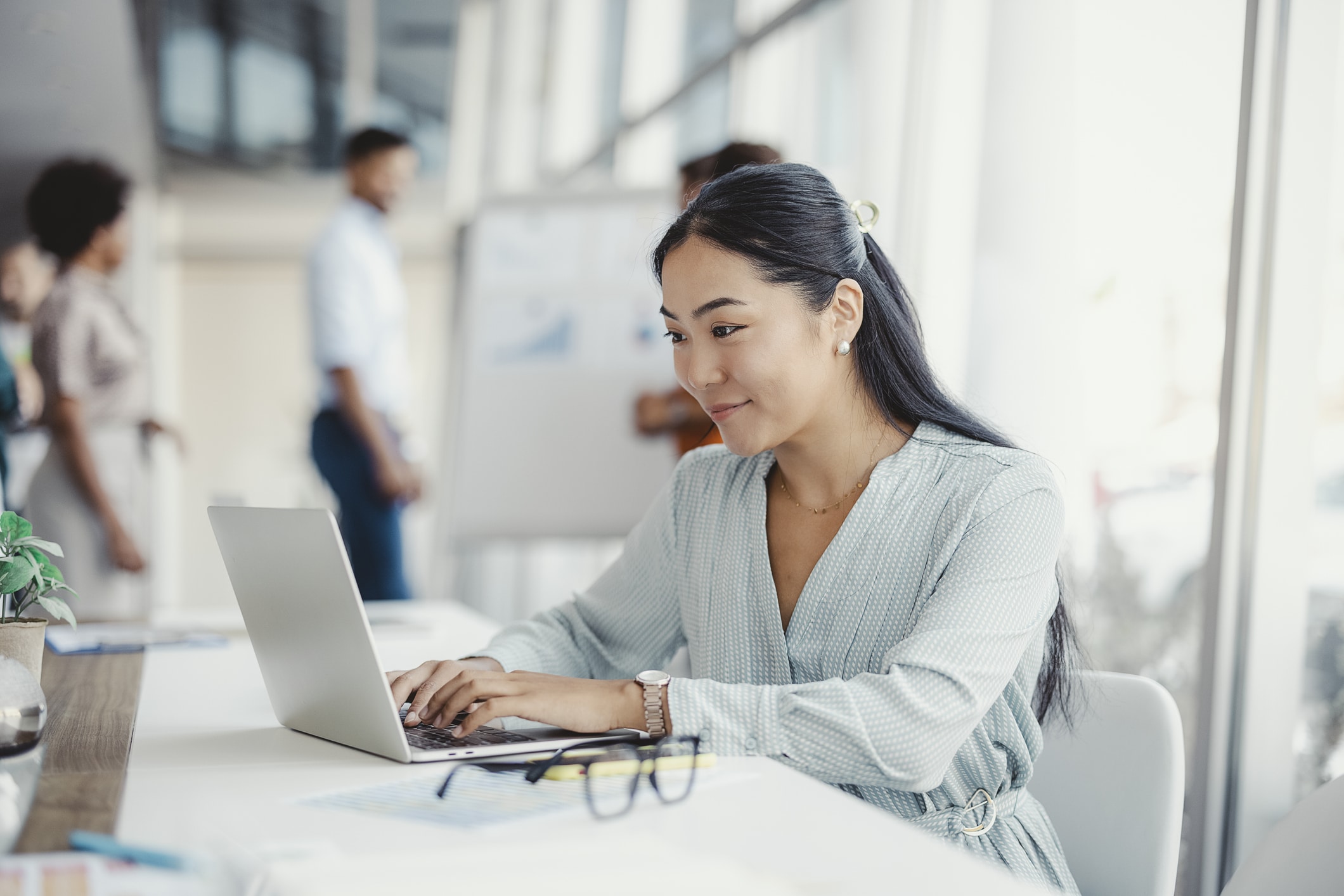
As artificial intelligence capabilities rapidly advance, their disruptive potential to transform organisations and societies is staggering. AI technologies are being infused across products, services, and operations – enhancing automation, predictive capabilities, and intelligent decisioning in ways that boost efficiency and unleash new opportunities.
However, this AI proliferation comes with significant risks if the technology is not used responsibly. Unintended biases, lack of transparency, vague accountability lines, sensitivity violations, and other ethical pitfalls could undermine trust and produce adverse consequences.
That’s why responsible artificial intelligence – grounded in core principles around accountability, fairness, inclusivity, reliability, privacy, and transparency – is critical for long-term success. Establishing a responsible AI policy ensures that AI systems are developed with integrity, helping to build customer trust in AI and maintain trust and reputation in AI-driven decisions.
The Core Principles of Responsible AI
While there is still a lack of global consensus around the responsible use of AI tools, Microsoft has emerged as a leader in defining and promoting ethical and responsible AI in the enterprise through its Responsible AI Standard. The latest version 2 outlines key principles that organisations should embrace:
Accountability
There must be human accountability for AI generated outcomes across the entire lifecycle – from design through to deployment and ongoing monitoring. Processes should enable auditability, interpretability of how decisions are made, and with adequate human oversight. For example, if a person was to prepare a budget in Excel and one of their formulas was incorrect resulting in inaccurate figures then the responsibility for the mistake lies with them. Similarly, if a person were to use incorrect information provided by AI, then the responsibility to check and correct it also lies with them. This principle reinforces trust in AI systems.
Fairness
Ensuring reliability and avoiding unfair bias are critically important. AI should be designed and used to equitably distribute opportunities, resources and information in ways that prevent unfair bias, discrimination or penalisation of individuals or groups. Proactive steps like representative data collection and applied fairness techniques should be taken.
Inclusivity
AI should be designed and used to benefit and empower everyone equitably, incorporating accessibility from the ground up. It also needs to agnostically accommodate diverse geographic/cultural circumstances.
Reliability and Safety
AI must be rigorously and functionally tested across diverse situations. Investigation and mitigation of potential risks to individuals, businesses and society is paramount, before it can be used as general practice, building trust and reputation in AI.
Privacy and Security
A responsible AI policy must prioritise privacy through intelligent data management practices like data minimisation, sensitivity classification, purpose limitation, and security in data collection/usage. Strong data governance frameworks and privacy protection measures are required, particularly when dealing with sensitive personal data used by AI.
Transparency
There must be traceability on how AI generated outputs are used. Model documentation should be created that clearly outlines AI strengths, limitations, intended use case, and training data allowances. Transparent communications help build customer trust in AI, ensuring users understand when human involvement is required.
Embedding Responsible AI Through Intelligent Data Management
Adhering to these principles of responsible AI requires robust intelligent information management strategies and capabilities that extend across the entire information lifecycle – from sourcing high-quality training data to monitoring model performance as well as ongoing improvement validation and regression.
By leveraging AI in the enterprise, like machine learning algorithms, small / large language models, cognitive services (such as natural language processing), and more, intelligent information management platforms can streamline and optimise key data preparation processes by leveraging responsible AI frameworks, including:
- Automatically finding, classifying and organising data repositories to ensure trusted data provenance.
- Identifying, anonymising, and protecting sensitive personal and confidential data to safeguard privacy.
- Applying data quality practices and cleansing processes to create AI-ready datasets that support building trust in AI through responsible use.
- Incorporating data traceability, versioning, and auditability of data flows by design into AI pipelines.
- Continuously monitoring and maintaining data quality to sustain accurate AI generated outputs over time.
The EncompaaaS Difference
At EncompaaS, we believe intelligent information management is the key responsible AI in the enterprise while fostering trust in AI systems and supporting ethical AI innovation.
Having embraced the Microsoft Responsible AI Standard v2 in our information management architecture, and incorporating it into our platform, EncompaaS enables organisations to build trust in AI systems by:
- Finding, enriching, organising and de-risking structured, unstructured and semi-structured data.
- Creating a trusted data quality foundation for AI-powered decision making.
- Allowing users to interact with their entire data corpus using simple questions, with AI selecting the right tool to provide fully auditable and explainable results.
EncompaaS redefines information management, enabling organisations to discover, understand, and manage enterprise data, without the need for complex technical understanding. Our AI-powered platform allows customers to interact with their entire data corpus through natural language queries. EncompaaS dynamically selects the right tool to provide the best answer, complete with full auditability, confidence levels, and reasoning. This includes the ability to configure prompts for AI models (such as GPT4) making decisions such as risk, ownership, sensitivity, over-retention, and more much quicker and easier to answer.
By embedding responsible AI into information governance, EncompaaS facilitates a responsible framework for information managers to review, agree, or correct AI-generated suggestions. This automated governance ensures alignment with organisational risk tolerance and trust, delivering clarity and accountability at scale.
To ensure transparency and to support human oversight of AI generated suggestions, EncompaaS presents details of the confidence levels for each suggestion along with the reasoning behind it.
EncompaaS also presents alternative suggestions above a certain (and fully configurable) confidence threshold, highlighting the confidence level separation between suggestions. This allows users to identify potential performance issues, “false positives”, as well as safety and privacy concerns, ensuring AI-powered decisions remain trustworthy, secure, and aligned with regulatory expectations.
Build Trust and Resilience Through Responsible AI
As AI’s influence continues to reshape our world, public trust in the technology will hinge on organisations proactively prioritising responsible artificial intelligence approaches that incorporate transparency and accountability. Ethical and responsible AI in enterprise requires robust governance frameworks, intelligent data management, and transparent decision making.
Establishing robust information management practices that uphold responsible principles will be imperative for building trust in AI capabilities that drive sustainable innovation into the future.
To learn more about how EncompaaS can help your organisation achieve data readiness in preparation for the responsible deployment of AI, contact us or book a demo.
Book a demo
Let's get started
Experience the Power of EncompaaS!
Submit this form to see EncompaaS in action with a demo from our information management experts.
Related Resources
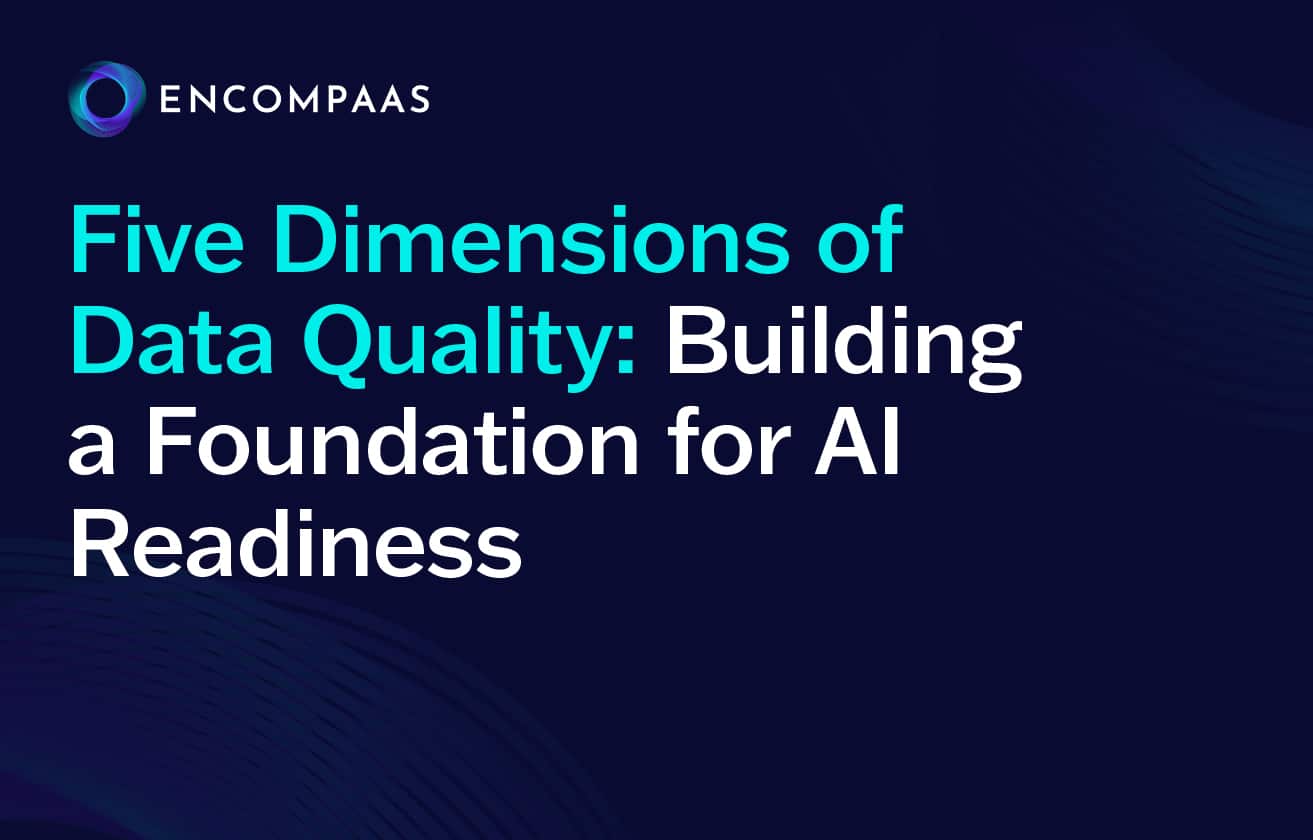
- Blog
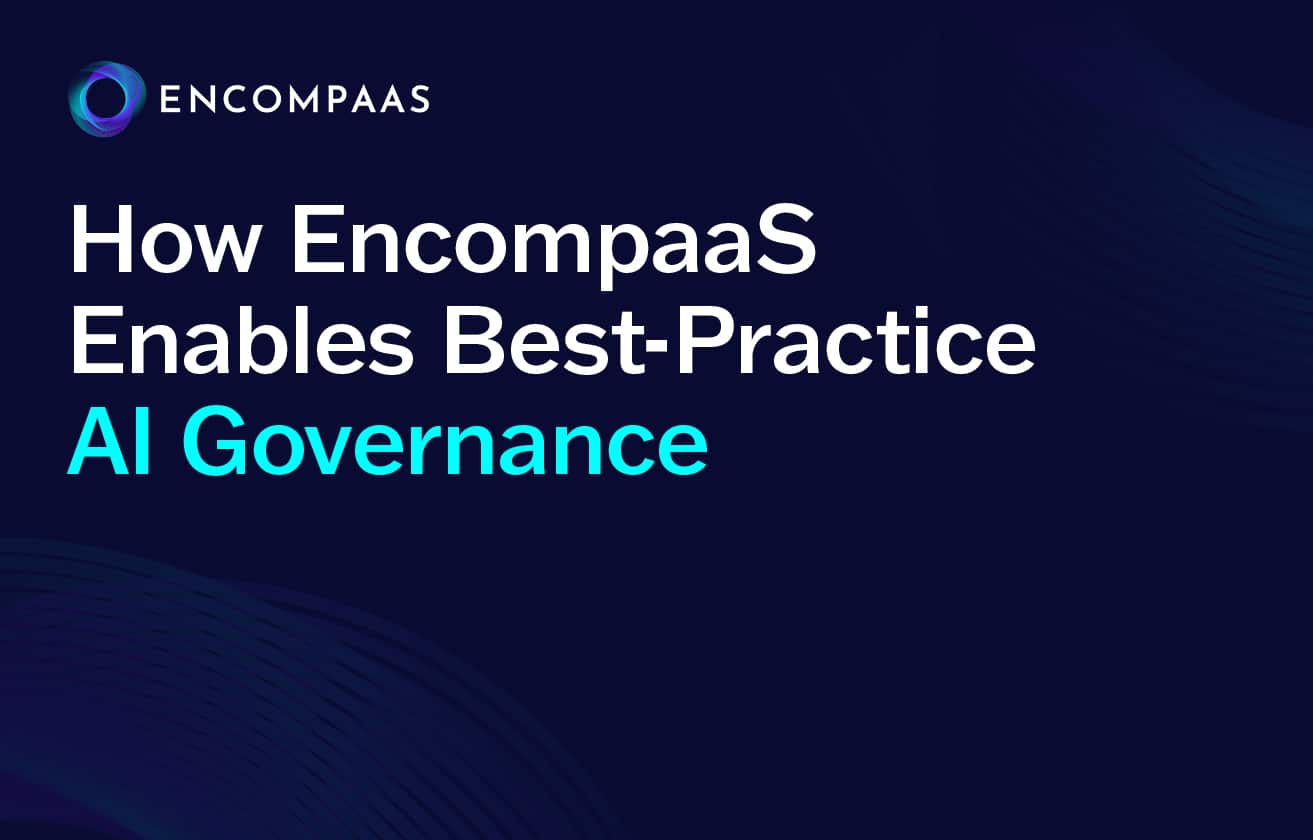
- Blog