Data Normalisation: The Unsung Hero of AI Readiness
Authored by EncompaaS - Apr 3, 2025
Share
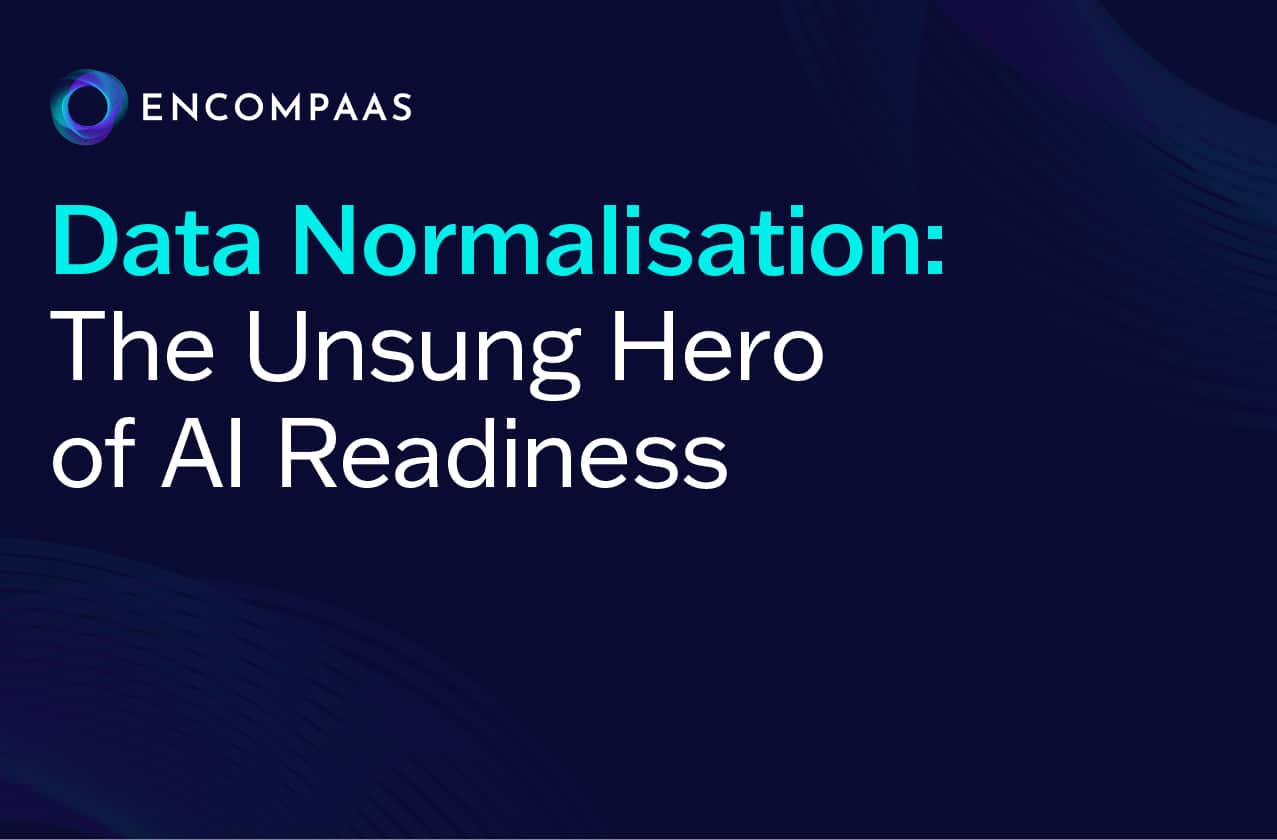
Today’s organisations increasingly view artificial intelligence as critical to their competitive strategy. Yet, in their urgency to harness AI’s potential, many overlook one fundamental truth: the quality of AI outcomes depends entirely on the quality and consistency of the data that feeds it.
According to Gartner, over 60% of organisations either lack AI-ready data or remain uncertain about their data readiness. Without effective data normalisation, enterprises face data inconsistencies and inaccuracies, directly impacting the reliability of AI models, the efficiency of model training and the ability to scale initiatives effectively.
As organisations across industries recognise the enormous potential and economic impact of AI, ensuring that data is properly normalised becomes essential.
The strategic role of data normalisation in enterprise AI
Data normalisation systematically transforms diverse datasets from multiple sources into a consistent, unified format. It ensures structured, semi-structured and unstructured data becomes fully compatible and consistent, enabling enterprises to seamlessly integrate it into AI and machine learning workflows.
Organisations should be aware of the differences between data normalisation vs standardisation:
Normalisation scales data to a specific range (often between 0 and 1), ideal for algorithms like neural networks for fraud detection in banking, predictive maintenance in manufacturing, or image recognition in healthcare.
Commonly used data normalisation techniques include:
- Min-max scaling: Adjusting data to a specific range, often between 0 and 1.
- Z-score normalisation (standardisation): Centres data around a mean of zero and standard deviation of one.
- Decimal scaling: Shifts decimal points to simplify large numeric values.
Standardisation adjusts data to have a mean of zero and standard deviation of one, useful for algorithms that assume a normal data distribution, such as linear regression. Financial services might use standardisation for predictive analytics or risk modelling, while government agencies could apply it in economic forecasting or statistical analyses, where assuming data normality improves accuracy.
Understanding when to apply data normalisation versus standardisation ensures the effectiveness of your AI use cases.
Enhancing AI outcomes through data normalization
Data normalisation directly shapes the effectiveness of AI initiatives, boosting accuracy, training efficiency and scalability. By transforming diverse datasets into a consistent format, enterprises can achieve powerful AI-driven results.
Firstly, normalised data creates balanced and unbiased inputs, enabling AI models to make more precise predictions and reducing the risk of skewed outcomes. When data is normalised, algorithms focus evenly across features, increasing reliability and accuracy.
Secondly, consistent data cuts training times dramatically. With less computational effort required, organisations can swiftly iterate and deploy their models, turning insights into actions faster.
Finally, scaling AI becomes simpler with normalised data. Organisations can integrate new datasets, quickly expanding their AI capabilities to new use cases or departments without risking data quality or compliance.
Prioritising data normalisation ensures enterprises not only optimise immediate AI outcomes but also build a solid foundation for agile, long-term growth.
Real-world examples of effective data normalisation
Organisations across industries rely on data normalisation to enhance AI-driven outcomes:
- Financial services: Banks use normalisation to standardise data for regulatory compliance and accurate fraud detection, reducing financial and compliance risks. For instance, EncompaaS helped a global insurer reduce data retention by 30%, significantly lowering storage costs and compliance risks through automated normalisation and governance.
- Manufacturing: Manufacturers normalise data from various sensors and systems to power predictive maintenance, leading to decreased downtime and costs. Using EncompaaS, a leading manufacturer automated warranty data management—discovering, enriching, and normalizing information across systems for real-time analytics and greater productivity.
- Healthcare: Healthcare organisations rely on normalised patient data to strengthen predictive models, improve diagnostic accuracy and meet stringent regulatory requirements such as HIPAA. Leveraging platforms like EncompaaS allows healthcare providers to rapidly integrate and normalise diverse data, improving patient care outcomes and regulatory alignment.
- Government: Governments rely on normalised data for regulatory compliance and streamlined data integration from multiple agencies. For example, a state government department used EncompaaS to automate data discovery and normalisation after agency amalgamation, unifying data from multiple systems, improving compliance and streamlining operations for future AI initiative
By prioritising data normalisation, organisations can drive greater accuracy, efficiency and scalable AI initiatives that deliver tangible business results.
Accelerating AI readiness through automated data normalisation
Relying on manual data normalisation often creates bottlenecks that drain resources, amplify risks and slow innovation. Automating this process turns data chaos into a clear pathway toward rapid AI adoption and competitive advantage.
The EncompaaS AI-powered information management platform tackles data complexity head-on, using advanced AI to automatically discover, classify, enrich and normalise structured, semi-structured and unstructured enterprise data. By proactively standardising diverse data sources, EncompaaS builds a reliable, high-quality foundation ready to fuel your AI initiatives.
The platform also dynamically aligns normalisation practices to specific AI use cases. This tailored approach enhances AI accuracy, enhances organisational agility and positions your enterprise to quickly adapt and respond to emerging opportunities and business demands.
Accelerate your AI journey with EncompaaS
Data normalisation is fundamental to AI success, transforming scattered, inconsistent data into a reliable, high-quality foundation.
Automating data normalisation with EncompaaS empowers enterprises to rapidly scale AI initiatives, enabling teams to innovate faster and with greater certainty in a competitive digital landscape.
By removing the complexity and manual effort from data normalisation, EncompaaS enables your organisation to confidently harness AI’s transformative potential, reduce risks and achieve superior business outcomes.
Request your EncompaaS demo today and see how data normalisation can unlock your organisation’s full AI potential.
Book a demo
Let's get started
Experience the Power of EncompaaS!
Submit this form to see EncompaaS in action with a demo from our information management experts.
Request a demo today
Related Resources
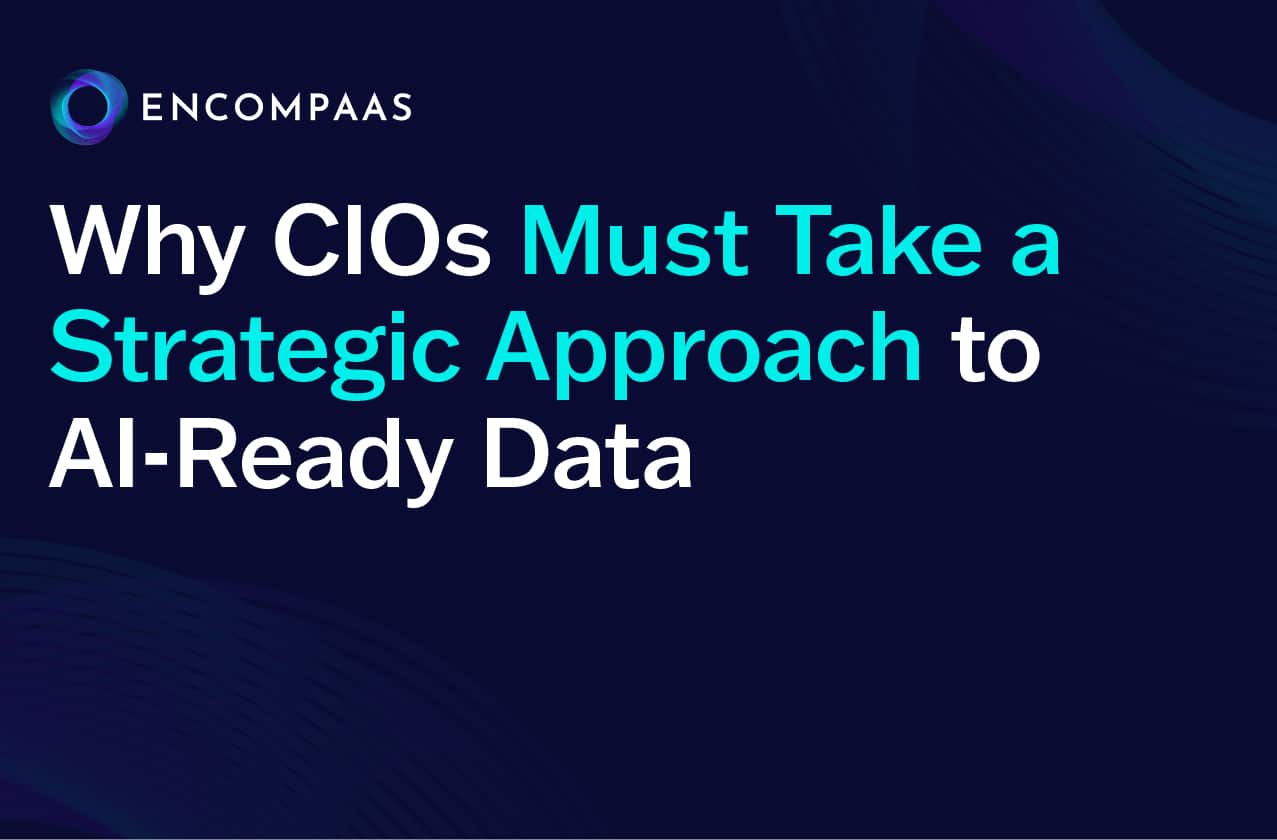
- Blog
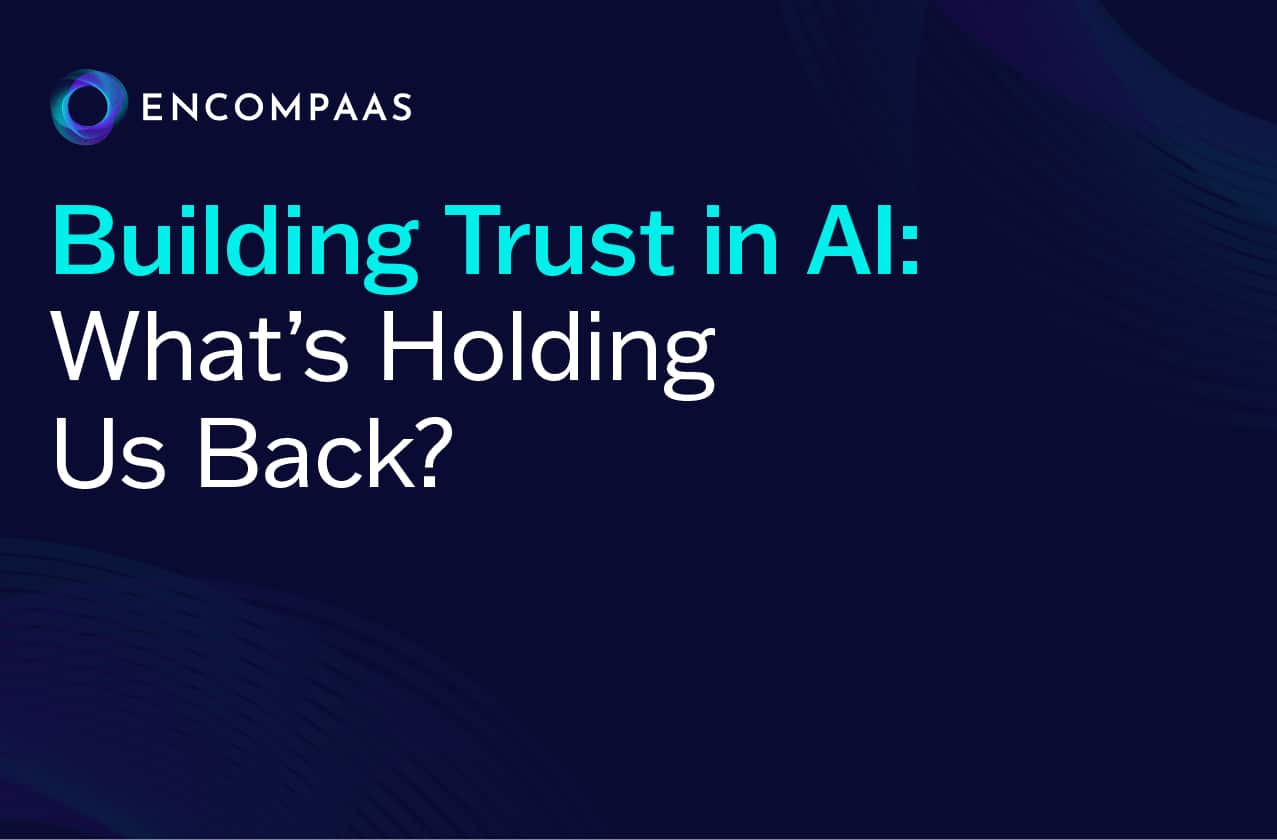
- Blog