Why AI Projects Fail: The Overlooked Role of Unstructured Data
Authored by David Gould - Mar 24, 2025
Share
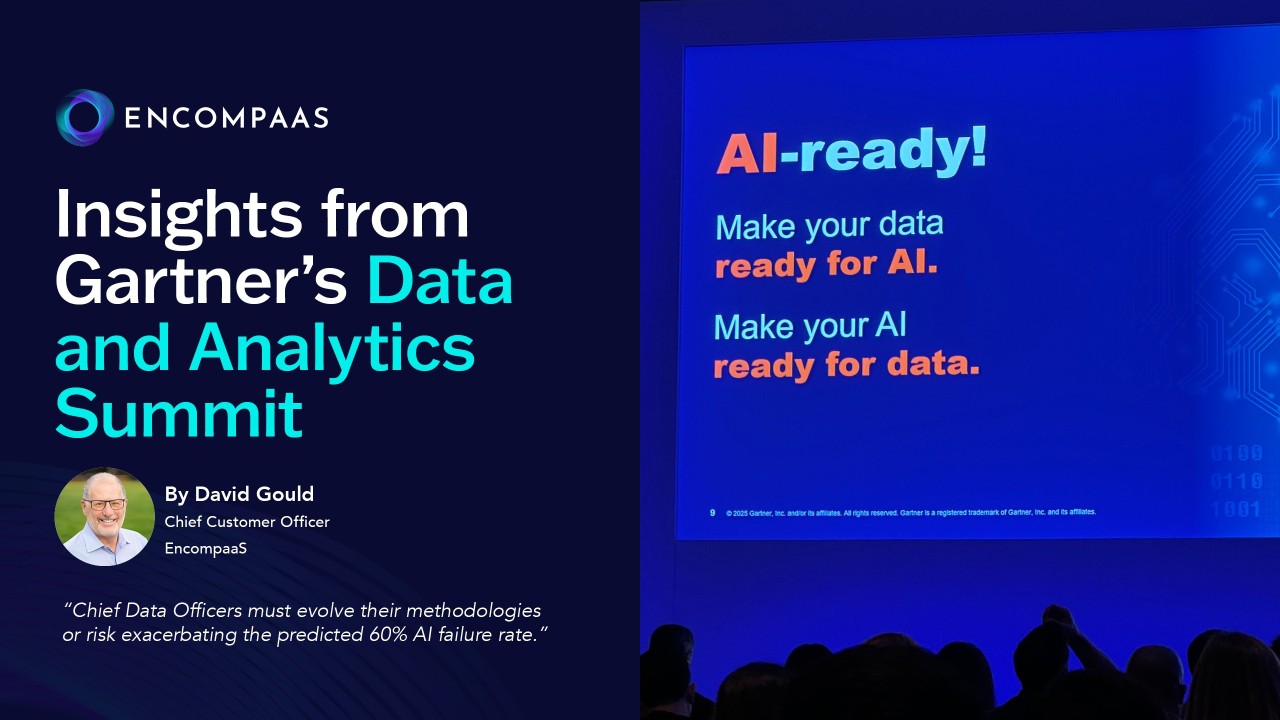
I’ve had the pleasure of attending this week’s Gartner Data and Analytics Summit in beautiful, sunny Florida. Unsurprisingly, the focus was on Generative AI (GenAI) and the ways enterprise data chieftains must evolve to harness one of the most transformative technological shifts in history.
What’s surprising? The dominant conversation at the event has revolved around structured data—even though Gartner itself reports that 90% of corporate data is unstructured. This disconnect highlights a fundamental challenge: businesses are applying structured data governance techniques to unstructured data, creating a ‘square peg in a round hole’ scenario when preparing data for AI.
The GenAI Readiness Gap
Gartner VP Analysts Gareth Herschel and Carlie Idoine delivered an opening keynote, “Scale Data and Analytics on Your AI Journeys,” emphasizing a crucial distinction: data quality—a concept rooted in structured data—is not the same as AI data readiness. In fact, Gartner predicts that over 60% of AI projects will fail by 2026 without an AI-ready data foundation.
At EncompaaS, we define AI-ready data as content that is organized, structured, and formatted for optimal AI model training. This involves discovering, classifying, and enriching unstructured content—often containing sensitive information like PII, PCI, and PHI—while filtering out redundant or duplicate data. Without this foundational work, enterprises risk feeding incomplete, biased, or non-compliant data into their AI models, leading to unreliable outcomes.
Applying the Wrong Governance Model
At the conference, I saw a recurring challenge that we encounter daily when speaking with enterprise data leaders: structured data governance methods are being applied to unstructured content, despite their fundamental differences.
Traditional data governance focuses on master data management (MDM), lineage tracking, and data quality metrics—an approach designed for structured databases. But unstructured content requires document cognition, contextual analysis, and AI-driven classification to ensure its readiness for GenAI. The bottom line? Chief Data Officers must evolve their methodologies or risk exacerbating the predicted 60% AI failure rate.
Rethinking Data Readiness for GenAI Success
If there’s one certainty about GenAI, it’s that treating data readiness as a structured data problem will lead to failure. As Gartner emphasized this morning, data governance and data quality do not directly translate to AI data readiness.
Organizations that continue to conflate ‘quality’ with ‘readiness’ will struggle to produce reliable, trustworthy, and valuable AI-driven outcomes. EncompaaS understands these challenges deeply and has built its expertise around unstructured content—helping organizations unlock their data’s full potential while ensuring compliance and governance at scale.
If your organization is navigating these challenges and wants to see how to make data work effectively for GenAI, visit our website or reach out to me directly. We can show you the pathway to AI success.
Book a demo
Let's get started
Experience the Power of EncompaaS!
Submit this form to see EncompaaS in action with a demo from our information management experts.
Related Resources
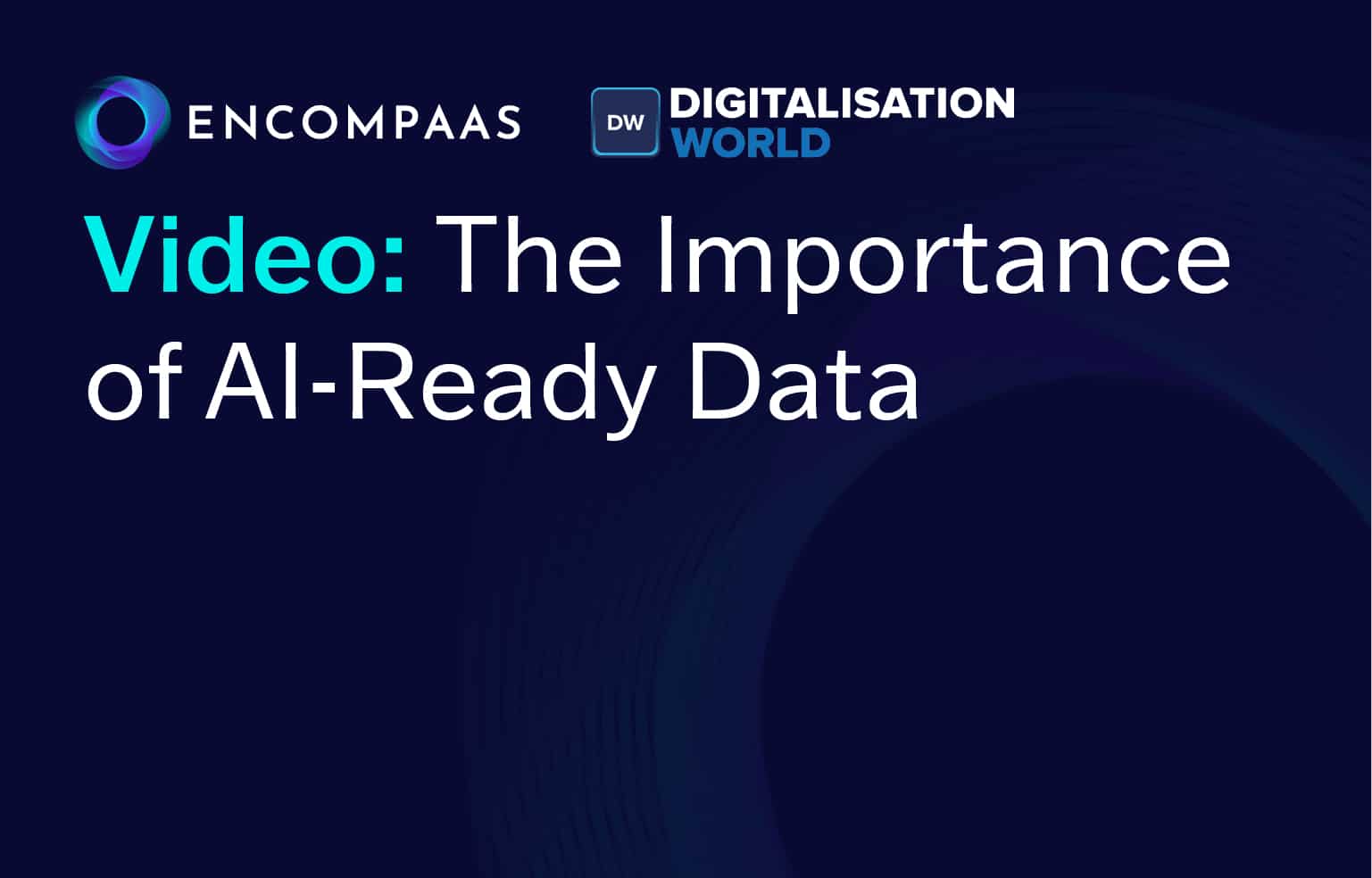
- Video
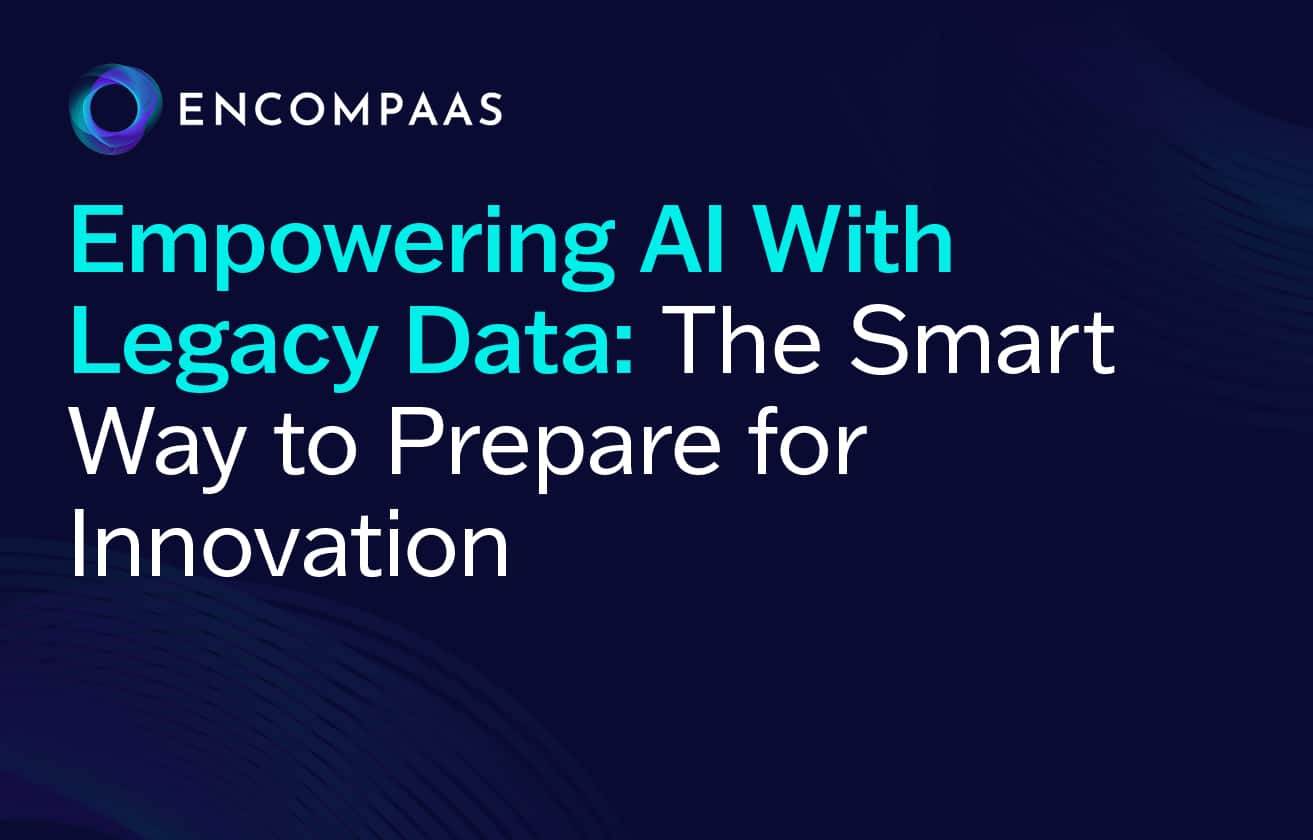
- Blog