AI and Privacy: How To Enhance Trust & Protect PII
Authored by EncompaaS - Feb 26, 2023
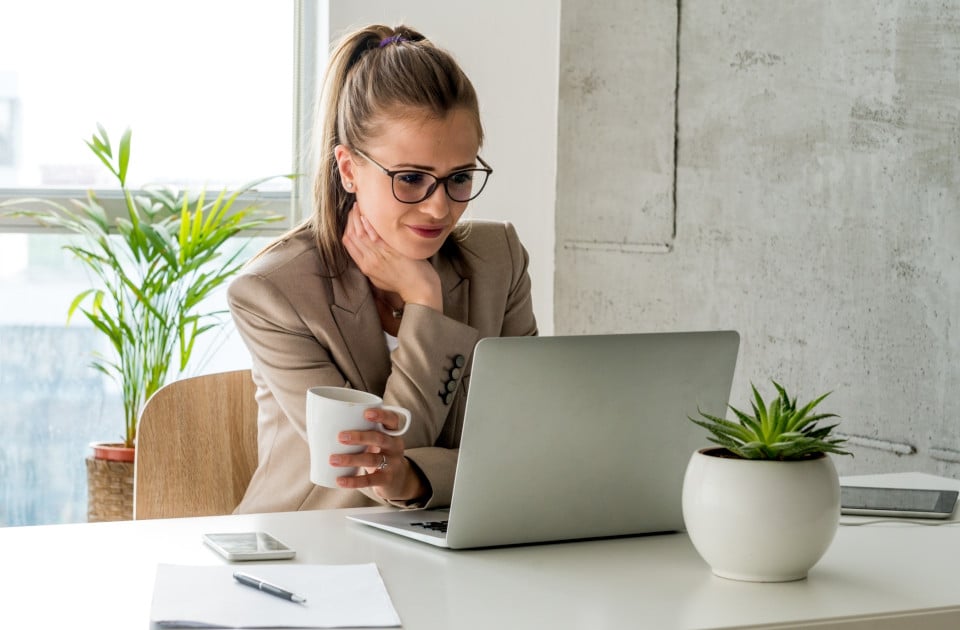
In our data-driven world, the trade-off between technological advancement and individual privacy often feels precarious. The capabilities of AI systems often hinge on vast quantities of personal information.
How do we reconcile the immense potential of AI with the urgent need to protect our most sensitive data and build trust between users and AI systems?
Let’s delve deeper.
Main takeaways from this article:
- As organizations increasingly incorporate AI into their operations, balancing technological innovation with protecting personally identifiable information (PII) is critical. Techniques such as data anonymization and secure AI development are essential strategies for maintaining this balance.
- The collection and analysis of data, whether structured, unstructured, or semi-structured, are fundamental to AI’s functionality. However, this raises significant privacy concerns, including unintended data exposure, data misuse, and vulnerabilities to cyberattacks.
- Addressing these issues requires a comprehensive approach to privacy that includes transparency, data minimization, and regular privacy impact assessments.
- Compliance with existing privacy laws like the GDPR and CCPA is crucial for organizations using AI, as these regulations set guidelines for data usage, consent, and consumer rights.
- Future privacy laws will further influence AI use, necessitating proactive compliance and privacy-focused design to reduce legal risks and build customer trust.
- Implementing strategies such as data anonymization, secure system development, and transparent data usage policies can help enhance privacy and trust in AI systems.
- EncompaaS offers a robust AI solution that enables businesses to leverage AI capabilities while upholding strict privacy standards, highlighting the importance of choosing the right AI tools to prioritize privacy.
AI data collection and analysis: A brief overview
To fully grasp AI data collection and analysis, it’s essential to first understand the difference between predictive and generative models.
Predictive AI leverages algorithms and models to predict future outcomes based on historical data. Think of it as a crystal ball, utilizing past patterns to speculate on forthcoming events.
Generative AI, on the other hand, doesn’t just predict – it creates. Using learned patterns from data, generative AI can produce entirely new instances of data.
For instance, this creative AI model can take a sketch and transform it into a photorealistic image or turn a melody into a symphony.
Both predictive and generative AI models depend heavily on ‘big data.’ Sophisticated algorithms develop more accurate predictions or generate more plausible creations when they access large datasets.
Data collection sources and methods
Data collection is at the heart of AI systems. But how is this data obtained? Let’s break down the various data types and collection methods:
- Structured data: Also known as quantitative data, structured data is organized into tables that can be easily understood by AI algorithms. An example would be a customer database with specific fields like name, email, and purchase history.
- Unstructured data: Qualitative or unstructured data is information that doesn’t adhere to a specific form. This could include reviews, social media posts, or audio recordings. Unstructured data can provide richer insights but requires advanced techniques to process and analyze.
- Semi-structured data: As the name suggests, this data lies somewhere between structured and unstructured. Examples include XML files or email data – information that doesn’t fit neatly into tables but comes with some organizational properties.
- Streaming data: This refers to data that is generated continuously by thousands of data sources, which could include GPS data or social media posts. It can be processed to enable real-time analytics and decision-making.
- Direct & indirect collection methods: Direct data collection involves actively seeking out data through surveys or interviews. Conversely, indirect collection might tap into existing data sources, like data from a customer relationship management tool or social media analytics.
Privacy concerns in AI systems
As artificial intelligence evolves, the handling and safeguarding of personally identifiable information (PII) becomes a growing concern. The integration of AI into databases containing sensitive information poses several privacy risks.
Let’s dig deeper into these risks and the challenges involved in ensuring data privacy within AI systems.
The inherent privacy risks in AI systems
AI systems pose several risks to the privacy of PII. These risks stem from both the immense amount of data they require and their complex algorithms. Here are some prevalent concerns:
- Unintended data exposure: While collecting and analyzing data, AI systems might inadvertently expose sensitive PII due to a lack of robust security measures or failure to anonymize such data.
- Data misuse: The misuse of data is another risk. Without explicit and informed consent from individuals, any use of their PII for purposes other than those initially stated constitutes misuse.
- Data breaches: AI systems, like any digital system, are vulnerable to cyberattacks. Hackers might exploit vulnerabilities within these systems to access and steal PII.
Challenges of ensuring privacy in AI systems
Securing PII within AI systems is not easy. AI operations and data flows are typically complex, making it challenging to implement effective privacy safeguards. Some of these challenges include:
- Complexity of AI algorithms: An AI system’s algorithm may be so complex that unintentional exposure of PII can happen, even with the best intentions to protect it.
- Data scale: AI systems often depend on vast amounts of data. The sheer scale of this data can make it harder to maintain consistent, comprehensive privacy protections.
- Evolving regulations: Legal and regulatory frameworks around data privacy are continually evolving, making it difficult to ensure compliance.
The role of comprehensive privacy legislation
Existing privacy laws like the General Data Protection Regulation (GDPR) and the California Consumer Privacy Act (CCPA) influence the deployment of AI systems and overall data management.
Considering the sensitive nature of the input data in AI systems, such legislation provides:
- Strict guidelines for how the data collected should be used and stored.
- A mandate on getting explicit consent from individuals prior to their data collection or usage in AI systems, as specified by GDPR.
- Provisions for consumers to opt out of data sales.
Future privacy laws could further define how organizations use AI, particularly in the handling of personal data. Therefore, adherence to these privacy regulations becomes a strategic advantage for cultivating loyalty in customers, protecting the business from expensive legal violations, and preventing any reputational damage.
Given the dynamic nature of data privacy legislation, it’s not viable for organizations to adopt a reactive approach.
Instead, to effectively stay ahead:
- Companies must proactively implement privacy measures to collect data.
- Consider the strategy of privacy by design when designing AI systems.
- Conduct regular privacy audits and promote data anonymization.
- Invest in advanced technologies that prioritize privacy when implementing AI solutions.
Privacy-conscious consumers are increasingly attracted to businesses that respect their data privacy and leverage AI solutions that offer the same commitment.
Strategies for enhancing privacy in AI systems
Now, let’s look at specific strategies that can be implemented to enhance privacy and build trust within AI systems.
1. Data anonymization and pseudonymization
For protecting privacy, techniques like data anonymization and pseudonymization must be used to mask PII and prevent individuals’ identification. They modify or encrypt original data to create non-identifiable data sets, which protect sensitive information while allowing for vital AI processing and analysis.
2. Secure AI system model development
When developing AI systems, certain security measures and risk assessments become paramount to safeguard collected data. These include:
- Privacy-preserving AI techniques: These methods help protect sensitive data during AI model training.
- Secure aggregation processes: They ensure that only trusted individuals or systems can access the data.
- SecureML: This system allows for scalable and privacy-preserving machine learning.
- A structured overview of techniques: This aids in identifying potential privacy vulnerabilities in AI systems, helping developers safeguard against them.
3. Transparent data usage policies
Create transparent policies on data usage to remove ambiguities about how your business should handle personal data. By clearly stating their data collection, processing, and minimization strategies, companies can respect consumer protection and privacy. This openness not only ensures legal compliance but also builds trust, which is key for forming successful customer relationships.
4. Regular privacy impact assessments
Implement regular privacy impact assessments (PIA) for AI applications to ensure risks associated with handling sensitive information and personal data are identified and mitigated in time. By consistently evaluating and adjusting your AI protocols, you can significantly enhance data protection and user trust.
5. Employee training and awareness
Complement AI with employee intelligence through tailored training programs. These programs should ensure that staff understand how AI operates and grasp the importance of privacy protection and regulatory compliance. Investing in employee training pays off in improved system usage, data handling, and regulatory adherence, creating a more effective digital workspace.
AI analytics process
Ensuring the privacy of user data at every phase of the analytics process is a crucial part of building trust in the AI systems of today and, more importantly, those of tomorrow.
Cleaning data with privacy in mind
Data cleaning in AI analytics involves scrubbing irrelevant or incorrectly formatted information and filling in missing data points, with a key focus on privacy protection. Using anonymization and pseudonymization techniques, sensitive personal identifiers can be effectively masked, drastically minimizing privacy risks.
Privacy-protected data processing
Once clean, your data undergoes structuring for useful analysis, necessitating robust security measures like encrypted databases for continuous protection. The application of data minimization principles ensures only necessary, relevant data points are processed and stored.
Analyzing data while upholding user privacy
In the final data analysis phase, AI models derive valuable insights while ensuring integrity through secure mechanisms against adversarial attacks. The adoption of techniques like differential privacy upholds the anonymity of data, safeguarding individual privacy even during its utilization.
Transforming data into knowledge
A transformative tool in data interpretation, AI works by scanning vast amounts of data in documents and identifying patterns and trends that were previously invisible to the human eye. Its algorithms sift through piles of structured and unstructured data, deciphering meaningful relationships and correlations.
However, the effectiveness of these insights doesn’t solely depend on the analytical prowess of AI; they’re equally dependent on the quality and diversity of the data being fed into the system. High-quality data ensures accuracy, while data diversity enhances the system’s understanding, facilitating unbiased, well-rounded predictions.
Profiling through AI: Benefits and risks
AI has the ability to create customer profiles by analyzing large amounts of data to find patterns and trends. This helps businesses offer personalized solutions, like ecommerce recommendations or educational pathways. Let’s look at some of the benefits of profiling through AI.
Benefits of AI-driven profiling
- Enhanced personalization: AI algorithms analyze customer data to offer tailored recommendations, improving the user experience.
- Efficient data analysis: AI can process vast amounts of data quickly, identifying patterns and trends that humans might overlook.
- Predictive analytics: AI’s ability to predict future customer behavior helps businesses anticipate needs and preferences.
- Operational efficiency: Automating the data analysis process saves time and resources, allowing businesses to focus on strategy and innovation.
However, AI intervention raises certain privacy concerns. Let’s look at some of the inherent risks associated with AI-driven profiling.
Risks associated with AI-driven profiling
- Privacy concerns: The collection and analysis of personal data raise questions about individual privacy. There’s a fine line between personalization and invasive surveillance.
- Data security: Storing large volumes of personal information makes companies a target for cyberattacks. A data breach can have catastrophic consequences for privacy and trust.
- Bias and discrimination: AI algorithms can perpetuate or even exacerbate biases present in the training data. This can lead to unfair profiling and discrimination against certain groups.
- Transparency and consent: Often, individuals are unaware of the extent of data collection and analysis. Businesses must ensure transparency and seek consent to build trust.
To address this, organizations need to carefully handle privacy issues by setting strict rules for data privacy in AI profiling methods. Protecting personal information should be a top priority, with measures like data encryption in place. It is also important to use other technologies to enhance the security of AI profiling projects.
Implementing AI operations with privacy in mind
When integrating AI technologies into your business operations, you must be careful not to compromise on privacy. Following the steps below can help ensure thoughtful and strategic planning.
Assess privacy risks
This first crucial step involves understanding the data your AI system will handle, foreseeing potential threats, and implementing stringent controls for protection. Any misuse could have serious repercussions, not only legally but also by damaging your brand’s reputation and consumer trust.
Choose the right AI solutions
Your choice of AI solution significantly impacts how well you can uphold privacy standards. Some solutions, like EncompaaS, offer advanced AI capabilities without sidelining privacy. EncompaaS allows you to leverage AI’s transformative potential while reassuring stakeholders that their PII remains secure.
Develop comprehensive data governance policies
Data governance in AI implementation serves as a crucial privacy shield by reducing potential data breaches and establishing a reaction framework for any violations. Your governance guidelines should prioritize data reduction, collection consent, transparent usage, and the rights of the data subject.
Trust and privacy in AI: The EncompaaS promise
At EncompaaS, we firmly believe in the harmonious blend of AI innovation and the rigorous protection of data privacy. Our mission is simple: to empower businesses with AI technologies that are not only groundbreaking but also deeply rooted in trust and compliance. Here’s how we stand by this commitment and what it means for you.
Our stance: A balanced approach to AI and privacy
We’re dedicated to a future where AI drives innovation without compromising data privacy. This balance is achieved through secure AI model development and transparent data usage policies, ensuring that every aspect of our platform respects and protects personal and organizational data.
How we achieve it: Advanced tools and practices
Beyond traditional measures, we utilize advanced tools to enforce comprehensive privacy measures, automating compliance and data protection in ways that set us apart. Our approach simplifies navigating complex regulatory landscapes, making compliance seamless and integrated.
Benefits for Our Customers
- Trust and confidence: Our commitment to privacy enhances trust in our AI solutions, giving you the confidence to innovate freely.
- Compliance assurance: With EncompaaS, adhere to the latest privacy laws effortlessly, reducing compliance risks and focusing on growth.
- Streamlined operations: Our automated tools free you from manual compliance tasks, allowing you to focus on strategic initiatives.
Embrace the future of AI with the assurance of unparalleled privacy and compliance. Discover the EncompaaS difference by exploring our Data Compliance & Privacy solutions. Ready to elevate your AI journey? Book a demo with us today and unlock the full potential of secure, compliant AI innovation.
Book a demo
Let's get started
Experience the Power of EncompaaS!
Submit this form to see EncompaaS in action with a demo from our information management experts.
Request a demo today
Related Resources
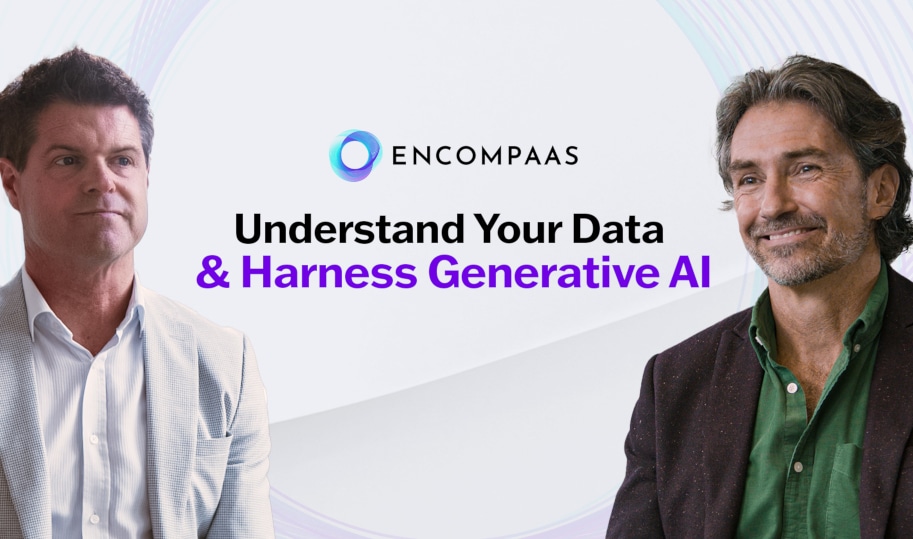
- video
Understand Your Data & Harness Generative AI
Jesse Todd (CEO, EncompaaS) and Jaimie Tilbrook (CPO, EncompaaS) delve into the critical challenges companies face in managing their vast data landscapes, emphasizing the importance of understanding what data is, where it is located, its context, and its value.
Learn More