What is AI data analytics? How it works & top use cases
Authored by EncompaaS - Feb 19, 2024
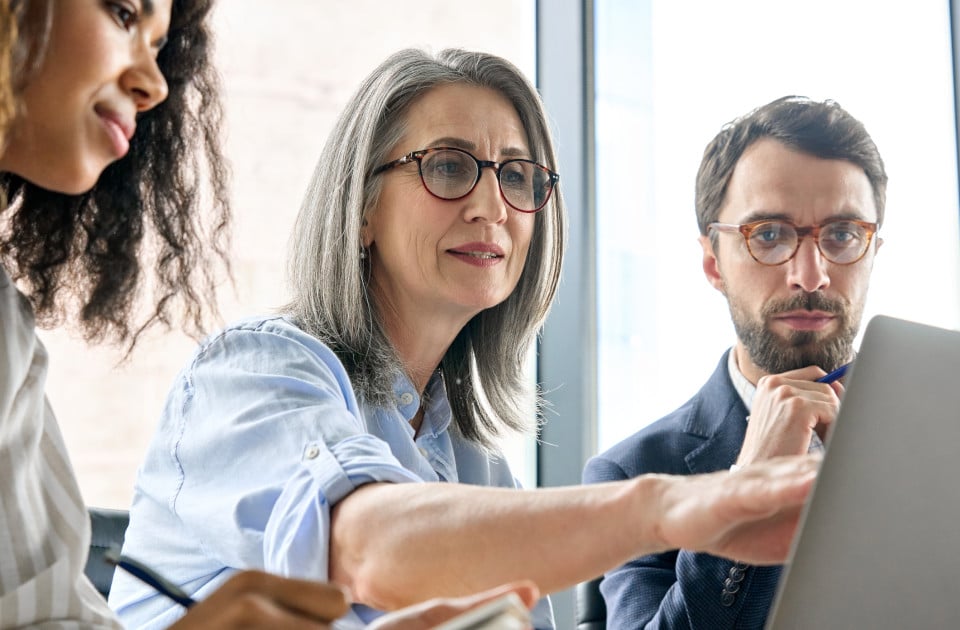
What if your business could predict future trends, personalize customer experiences, and make data-driven decisions faster than ever before? What if you could harness the power of immense data volumes and turn them into actionable insights with unprecedented accuracy? This isn’t science fiction – it’s the transformative potential of AI data analytics.
In this article, we’ll explore this innovative field in depth. From decoding complex algorithms to uncovering real-world applications, join us as we discuss AI data analytics and explore its top use cases across various industries.
Main takeaways from this article:
- AI data analytics integrates artificial intelligence technologies, including machine learning, deep learning, and NLP, to process data, generate insights, and make data-driven decisions.
- AI in data analytics streamlines data preprocessing enables prediction of future trends, processes large volumes of unstructured text data, and visualizes data dynamically and interactively.
- Data analysts and data scientists harness AI data analytics for better and quicker decision-making, scalability, predictive analysis, real-time data processing, and cost reduction.
- Despite its benefits, AI data analytics comes with challenges like ensuring data privacy and security, handling model complexity, ensuring data quality and availability, tackling skill gaps, and integrating and scaling solutions.
- Key applications of AI data analytics encompass customer behavior analysis, financial forecasting, fraud detection, healthcare diagnostics, patient care optimization, and supply chain management.
- EncompaaS, with its robust AI data analytics capabilities, helps automate data classification, analysis, and reporting, equipping businesses to leverage AI for strategic advantage.
What is AI data analytics?
AI data analytics, at its core, is about utilizing artificial intelligence technology to extract useful insights from vast data sets. It’s a practice that employs algorithms, computational intelligence, machine learning models, and other AI methods to convert raw data into actionable, value-rich information.
The distinguishing factors of AI data analytics
While traditional data analytics relies on manual interaction and pre-set rules to analyze data, AI data analytics introduces a layer of intelligence that permits self-learning and adapting to trends in real-time.
The key difference lies in AI data analytics’ capacity to handle massive data sets, untangle complexities, and deliver high-speed results without significant manual intervention, unlike its traditional counterpart.
Consider this. You’ve been analyzing trends in customer behavior using a traditional data analytics system. While useful, the process has been largely manual and lengthy, and the outcome is often delayed, so it might even turn out to be irrelevant.
Now, let’s introduce AI into the equation. The AI system can quickly grasp millions of data points, uncover hidden patterns and trends, and deliver the information just when you need it the most—all this in a fraction of the time the manual process would take.
AI’s role in the evolution of data analytics
Artificial Intelligence has transformed the way data is processed, making it possible to gain deeper insights from the overwhelming volumes of data generated every minute.
With machine learning algorithms, AI systems can learn from past data patterns, refine their data analysis process, and better predict future trends. Deep Learning, a subset of AI, has, in a sense, magnified this learning capability, empowering systems to learn from experiences and interpret new data based on what they’ve learned.
Both these technologies, foundational to AI, imagine a future where data analytics will be more about strategic thinking and less about slogging through massive data sheets.
How AI data analytics works
The triad of AI data analytics
- Machine learning (ML): This is the base that empowers AI to learn from data without explicit programming. ML algorithms seek patterns within data, learning and adapting over time to improve predictions or data-driven decision-making.
- Deep learning (DL): A subtype of ML, deep learning utilizes artificial neural networks with multiple layers (thus ‘deep’) of learning. Its remarkable capacity to process vast data sets and carry out complex commands sets it apart.
- Natural language processing (NLP): In the world of unstructured data, NLP shines by enabling computers to understand, interpret, and generate human language. It helps not only in text mining but also in sentiment analysis, machine translation, and more.
The process: From data collection to insight generation
AI data analytics isn’t just about having the right technological tools—it’s also about having a clear and structured workflow in place. Here’s what a typical AI data analytics process may look like.
- Data collection: This is the initial and, arguably, one of the most crucial steps. Whether it comes from databases, sensors, social media posts, or other sources, vast quantities of structured and unstructured data are harvested. But remember, quality trumps quantity—poor data will only lead to faulty insights.
- Data preprocessing: Raw data isn’t usually in a ready-to-analyze format. It may have irrelevant variables, outliers, or missing values that can skew your analysis. So, the data is cleaned, transformed, and prepared to guarantee it’s in the right state for AI data analysis.
- Modeling and analysis: At this stage, AI and machine learning algorithms are applied to the preprocessed data. Depending on the goal—whether that’s data clustering, classification, or regression—it’s here these algorithms churn the data and search for patterns.
- Inference and decision-making: Valuable insights are drawn from the model’s results. AI data analysis tools often visualize these findings using dashboards, making them easily digestible and actionable. These insights, whether predictive or descriptive, help businesses make informed, data-driven decisions.
Here’s a bird’s—eye view of the AI data analytics process. Each stage is important and plays its part in transforming raw data into valuable, actionable insights.
Key components of AI data analytics
Let’s delve into the intricacies of AI data analytics by examining its major components. Each part plays a crucial role in harnessing intelligent data insights from vast volumes of raw data. It’s through this blend of components that AI data analytics truly shines.
1. AI in data preparation and cleaning
AI significantly automates data preprocessing, which traditionally is a labor-intensive and time-consuming phase in data analysis. AI algorithms can automatically detect and handle missing or abnormal data, reducing errors and improving efficiency.
2. Predictive analytics through AI
AI brings an unparalleled edge to predictive analytics. By analyzing historical data and identifying patterns, AI-based models can predict future trends and behaviors with remarkable accuracy. This predictive power can be leveraged across numerous sectors, from finance to healthcare, for informed decision-making.
3. Natural language processing (NLP) through AI
NLP, a subset of AI, has transformed the way we analyze unstructured text data. NLP can comprehend human language, sentiments, and context within the data. This ability allows organizations to gain insights from previously untapped resources like social media feeds, customer reviews, and more.
4. Visualization tools powered by AI
Data visualizations become more dynamic and interactive through AI tools. These tools can automatically generate clear and intuitive graphics, dashboards, and reports from large and complex datasets. They not only simplify data interpretation but also make insights accessible to a broader audience within an organization.
Benefits of AI data analytics
As we delve into the remarkable world of AI data analytics, let’s look at the tangible benefits that this technology brings. AI data analytics has emerged as a game-changer, propelling businesses with enhanced decision-making, scalability, predictive abilities, real-time insights, and significant cost reduction.
1. Enhanced decision-making and efficiency
AI data analytics integrates machine learning and predictive modeling capabilities that can rapidly process and analyze huge data sets. This accelerates decision-making processes by offering actionable insights quicker than traditional methods.
It also reduces the manual effort needed in data interpretation, hence improving efficiency. Furthermore, it leaves little room for human error, moving towards more dependable, data-driven decisions.
2. Scalability and improved accuracy
As organizations grow, the complexity and size of their data increase. AI data analytics is scalable, managing large volumes of data with high accuracy while maintaining data integrity and consistency even if the business expands significantly.
The use of AI also reduces errors in data analysis, enhancing the overall precision of findings.
3. Predictive analytics and personalization
AI is a game-changer for predictive analytics. It systematically uses historical data to forecast future trends, helping businesses anticipate the next developments and personalizing customer experiences.
Companies can now tailor their services and products, targeting customers based on their predicted behaviors, preferences, and needs.
4. Real-time data analysis
With AI data analytics, businesses can analyze data in real-time. Immediate insights are especially critical when dynamic responses are essential, such as intervening timely in a critical operational issue or responding promptly to a customer’s query.
This continuous feed of insights helps firms stay agile, informed, and responsive to change.
5. Cost reduction
A significant advantage of AI data analytics is its cost-efficiency. The ability to automate processes and optimize resource allocation can significantly lower operational costs. AI can handle repetitive, mundane tasks faster than humans, leaving staff free to concentrate on more complex duties.
Plus, the use of accurate data insights for strategic decision-making can lead to improved organizational performance and cost savings in the long run.
Challenges with AI data analytics
While AI data analytics yields remarkable benefits, it’s only fair to acknowledge that it’s not without its challenges. Let’s delve into some of the obstacles faced in implementing AI in data analytics.
- Data privacy and security: Ensuring the protection of sensitive information while using AI algorithms.
- Complexity and interpretability: Managing the complexity of AI models and making their findings understandable to non-experts.
- Data quality and availability: Maintaining high-quality data inputs to avoid “garbage in, garbage out” scenarios.
- Skill gap: Addressing the shortage of skilled professionals capable of designing, implementing, and interpreting AI data analytics.
- Integration and scalability: Integrating AI analytics tools with existing systems and scaling solutions to meet organizational needs.
Top use cases of AI data analytics
1. Marketing and sales
With AI data analytics, businesses can delve deeper into customer behavior analysis to create highly personalized marketing and sales strategies. The technology allows companies to gather data from various sources, analyze the collected information, and gain critical insights into what piques customer interest and drives purchasing decisions.
As a result, businesses can tailor their marketing strategies to meet consumer expectations and demands, improving their bottom line.
2. Banking
AI data analytics is a game-changer for financial forecasting and fraud detection. Traditional methods often struggled to accurately identify patterns in enormous datasets. However, AI’s capacity to quickly process vast amounts of data enables it to unearth trends and spot anomalies that could indicate fraudulent activity.
This translates into more reliable forecasts and heightened security measures, increasing trust among clients and stakeholders alike.
3. Healthcare sector
AI data analytics plays a vital role in healthcare, especially in diagnostics and patient care optimization. AI systems can read and interpret medical imaging at impressive speeds, contributing to faster, more precise diagnoses.
Simultaneously, by analyzing patient data, these systems can suggest the most effective treatment options, ensuring each patient receives personalized care.
In this manner, healthcare providers can address health issues promptly, enhancing patient outcomes and satisfaction rates.
4. Manufacturing industry
AI data analytics is creating waves of change in the manufacturing industry. Through data interpretation, it’s now easier than ever to optimize the supply chain and perform predictive maintenance.
This technology can anticipate future market demand, facilitating accurate inventory planning, and can predict machinery malfunctions before they occur, mitigating downtime.
EncompaaS and AI data analytics: Powering enterprise strategies
In today’s data-driven landscape, AI data analytics has become an essential tool for unlocking insights and driving strategic decision-making. The ability to rapidly process, analyze, and extract value from vast amounts of data provides organizations with a significant competitive edge.
This is where EncompaaS excels, offering a sophisticated platform that seamlessly integrates powerful AI data analytics capabilities. EncompaaS empowers businesses to move beyond simple data management and into the realm of true data-driven transformation.
EncompaaS leverages the power of AI to deliver a range of intelligent data management solutions:
- Automated data classification and analysis: Streamline the organization and understanding of large datasets with precise classification and in-depth analysis powered by advanced AI algorithms.
- AI-driven reporting: Generate comprehensive, readily understandable reports that uncover trends, anomalies, and actionable insights within your data.
- Intelligent data management: EncompaaS integrates Azure OpenAI for search and auto-classification. This foundation is then augmented with specialized tools for a holistic, AI-enhanced approach to refining your data ecosystem across cloud and on-premises repositories.
- Unlocking hidden value in complex documents: Go beyond basic analysis with AI that examines contracts, agreements, and other multifaceted documents. Uncover micro-details for risk assessment and competitive opportunities.
EncompaaS stands apart by prioritizing data quality as the foundation for any successful AI initiative. AI outcomes are only as reliable as the data they are built upon. This commitment to quality, coupled with a flexible platform that adapts to the latest AI advancements, delivers tangible business outcomes.
Ready to step into the future with AI data analytics? Why not learn more about EncompaaS and explore its capabilities firsthand? Book a demo now to start your journey.
Book a demo
Let's get started
Experience the Power of EncompaaS!
Submit this form to see EncompaaS in action with a demo from our information management experts.
Request a demo today
* indicates required field
By submitting this form, you consent to be contacted by a member of our team by email or phone. You can unsubscribe from our communications at any time via the unsubscribe option in the email received. For more information on how EncompaaS uses your data, please refer to our Privacy Policy.
Related Resources
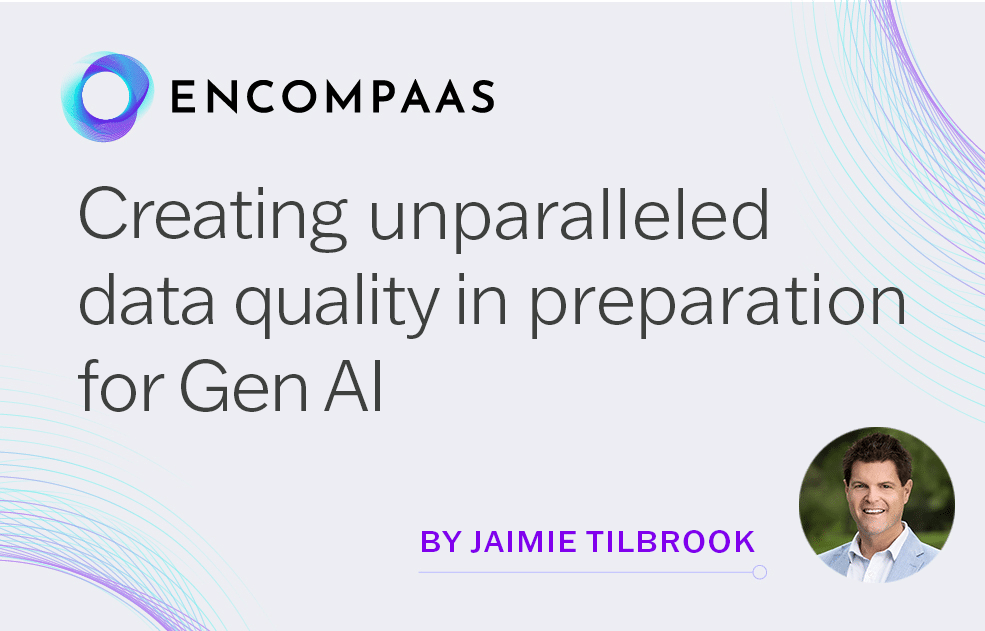
- blog
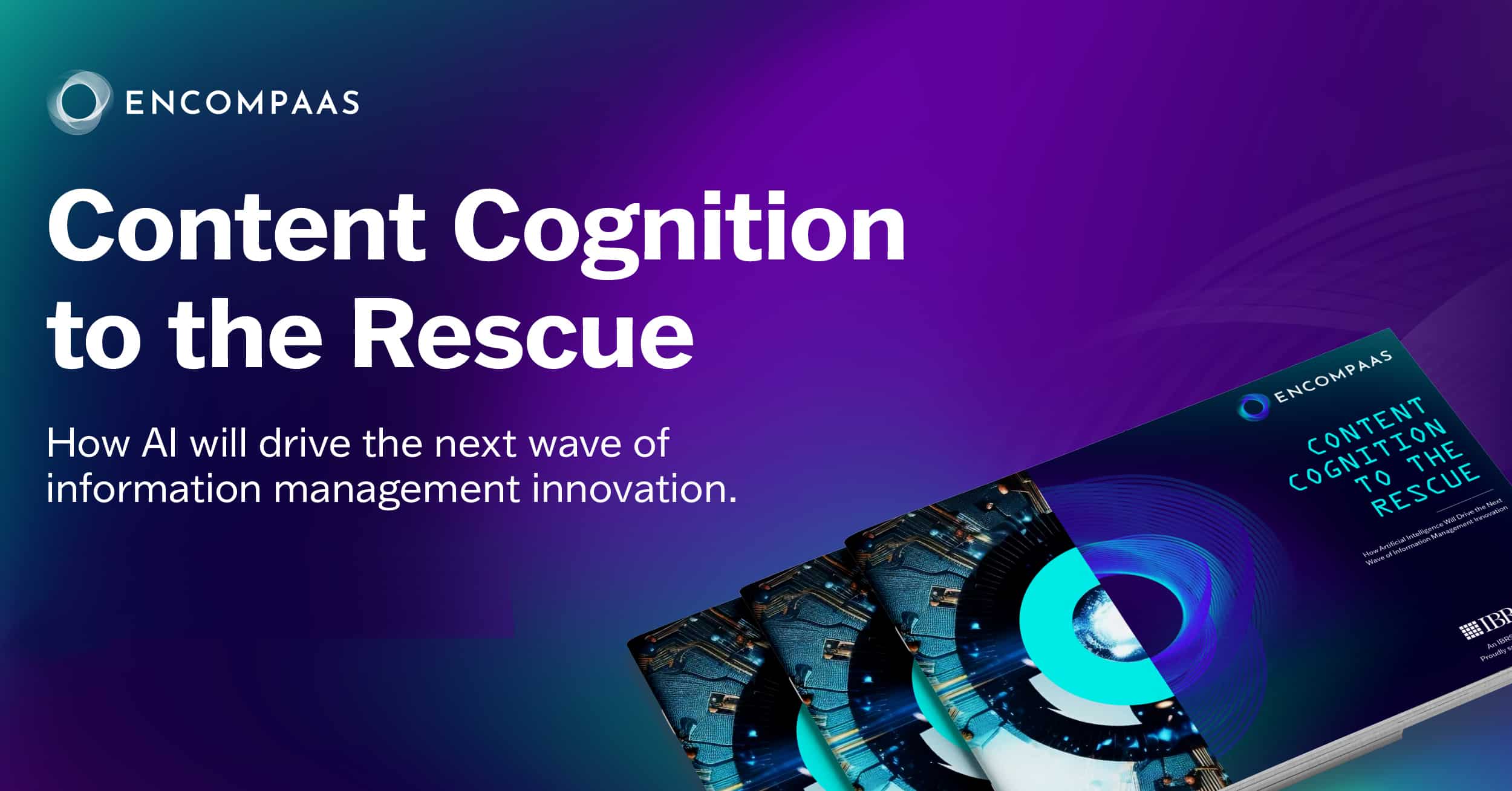
- press
‘Information Hyperinflation’ Challenging Ability of Australian Enterprises to Maintain Privacy and Security Governance, According to New Study
Hundreds of Information Management Professionals Surveyed in IBRS Report Sponsored by EncompaaS Confirm Growing Information Overload; AI-Based Tools Seen as Both Accelerating Problem and as Potential Solution
Learn More