Managing Unstructured Data: Best Practices & Tips
Authored by EncompaaS - Jul 8, 2023
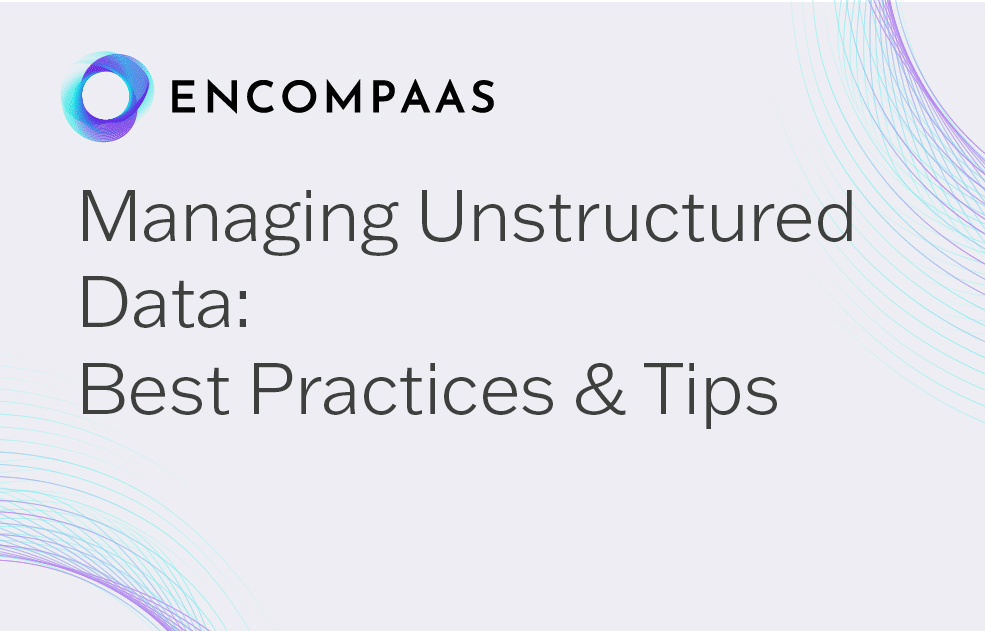
Unstructured data is growing exponentially. This surge is challenging organizations worldwide to harness its potential effectively. Unstructured data abounds in emails, social media feeds, videos, and more and presents a somewhat untamed frontier. While it carries powerful insights, navigating this vast landscape calls for novel approaches and tools.
This guide will focus on bridging that gap by discussing strategies and solutions for successful unstructured data management and utilization.
Main takeaways from this article:
- Unstructured data forms a significant portion of enterprise data, which, if properly managed, can generate valuable business insights.
- Key challenges in managing unstructured data include volume, variety, and veracity, with implications on data accessibility and compliance.
- Effective management of unstructured data involves strategies such as data discovery, classification, enhancement with metadata, robust data governance, and leveraging AI and machine learning for data analysis.
- Organizations should focus on continuous improvement in data quality, scalability in storage, and adoption of flexible data management solutions to support the growth of unstructured data and evolving business needs.
- EncompaaS’ platform enhances unstructured data management by leveraging AI and machine learning capabilities, highlighting how technology can streamline and optimize these processes.
What is unstructured data?
Unstructured data refers to information that lacks a pre-defined format or organization, making it challenging to process and analyze using conventional database systems. Examples include text files, videos, images, and social media content. This data type is rich in information but complex to utilize effectively.
Unstructured vs. structured data management
When it comes to managing data within an organization, there are considerable differences between structured and unstructured data. Understanding these dissimilarities is crucial for implementing effective management strategies.
Let’s delve into this:
Existence of a defined schema
Structured data exists within a predefined schema. This means it can be neatly organized in relational databases, making it easier to manage, search, and analyze. This feature lets structured data fit conveniently within spreadsheets and database tables. It’s akin to a well-organized library where each book has a specified place.
In contrast, unstructured data lacks such schema or format, rendering it more complex to store, process, and analyze. It resembles a heap of books without a classification system.
Data categorization
In structured data management, categorizing and retrieving data is simplified by the very nature of its structure. Information is easily classified based on parameters like date, number, and category.
However, unstructured data, consisting of emails, social media posts, videos, and other forms, does not lend itself to easy categorization or retrieval. This demands sophisticated tools and techniques to handle.
Processing and analysis methods
Standard programming tools can efficiently process structured data. This simplicity extends to data analytics, too, where structured data processing is straightforward using conventional data analytics tools.
However, the story is different with unstructured data. It requires advanced technologies, such as AI and machine learning, to interpret and analyze.
Storage requirements
Thanks to its inherent organization, structured data tends to take up less storage space. Conversely, unstructured data, due to its volume and variety, necessitates scalable, flexible, and secure storage solutions.
Considering these disparities can help devise optimized data management strategies for both structured and unstructured data, thus enabling a more harmonious data ecosystem within your organization.
The significance of unstructured data
Despite being more complex and challenging to manage, unstructured data holds immense potential for organizations. It acts as a goldmine of insights that, when harnessed properly, can drive informed business decisions, encourage innovation, and provide a competitive edge in today’s intricate marketplace.
To understand this better, let’s delve into how unstructured data can be a game changer:
- Customer insight: Unstructured data often includes customer feedback, surveys, social media comments, emails, and more. Analyzing this type of data can help you understand customer behavior, preferences, and sentiments, allowing you to tailor your products or services accordingly.
- Trend identification: Timely analysis of unstructured data can highlight emerging trends in your industry, helping your business adapt and always stay one step ahead.
- Decision-making: Businesses can use this information to drive strategic decision-making by converting unstructured data into structured data. It helps shed light on patterns, correlations, and anomalies that otherwise might go unchecked.
- Risk management: Unstructured data can also play a significant role in risk management. It can provide insights into potential risks and help devise strategies to mitigate them.
- Innovation: Unstructured data can fuel innovation by providing unique insights that can lead to the development of new products, services, or business models.
Challenges in managing unstructured data
Managing unstructured data presents unique hurdles primarily influenced by its massive volume, immense variety, and integrity challenges. Understanding each of these challenges is crucial for businesses to devise effective strategies for their data management endeavors.
Let’s dive into each of these obstacles:
- Volume: The sheer amount of unstructured data being produced each day is staggering. From emails and customer feedback to social media posts, businesses find themselves awash in a sea of data. This growth in data volume is exponential, only making management trickier. The challenge here lies in not only storing this massive data but also retrieving and analyzing it timely for productive purposes.
- Variety: Unstructured data comes in many forms—text documents, images, videos, emails, and even audio files. The inconsistency and varied nature of this data complicate categorization, analysis, and processing tasks. For businesses, ensuring that this varied data is compiled and stored in a manner that allows easy retrieval can be a daunting task.
- Veracity: Veracity refers to the reliability and quality of data. With unstructured data, establishing veracity can be challenging. The lack of structure and standardization poses difficulties in validating the accuracy and consistency of the data. Issues like duplication, redundancy, and inaccuracy can affect the credibility and usefulness of the data.
These challenges have significant implications for businesses in terms of data accessibility, analysis, and compliance. Let’s discuss these factors in detail:
- Data accessibility: The circumstances caused by these challenges often lead to data silos. As a result, crucial information becomes trapped in isolated systems or formats, making it inaccessible to users who need it. This negatively impacts operational efficiency and slows down decision-making processes.
- Data analysis: The volume, variety, and veracity issues make it difficult to perform comprehensive data analysis. Traditional data analysis techniques may not be suitable for unstructured data as they don’t allow for in-depth insights into the content or context of the data. This means organizations could miss out on valuable business insights.
- Data compliance: Regulatory compliance is becoming increasingly critical in the digital age. Failing to manage unstructured data effectively can lead to breaches in regulatory compliance. Businesses may struggle to identify and protect sensitive data, which could result in legal repercussions and damage to the organization’s reputation.
Best practices for unstructured data management and analysis
Now that we’ve identified the challenges let’s delve into actionable strategies for effectively managing and analyzing unstructured data. Follow these best practices to turn your data into a successful asset.
1. Initial data assessment and discovery
To effectively manage unstructured data, organizations must initiate the process with an initial assessment and discovery phase. This step is arguably one of the most critical, as it establishes the foundational understanding of the scope and nature of the unstructured data within your organization.
Let’s explore why initial data assessment and discovery are so important for unstructured data analysis:
- Determine the scope: This initial phase helps you ascertain the extent of your unstructured data. Just how much data are we talking about here? It’s essential to quantify the scale, which can range from a few gigabytes to potentially multiple petabytes. The volume of data directly impacts your storage, processing, and analysis strategies.
- Identify the type: Unstructured data isn’t limited to text documents. It includes emails, social media posts, images, audio files, video files, and more. Understanding these different types is key to laying out an effective management structure.
- Classify based on sensitivity: Not all data are created equal. Certain unstructured data files may contain sensitive information that requires additional security measures. As part of the initial assessment, you should identify and categorize sensitive data to ensure proper handling and compliance.
- Data relevancy: The discovery phase also helps determine the relevance of the various data types. This is crucial because not all data may be valuable or relevant to your business objectives. Distinguishing between useful and useless information can save time, resources, and storage space.
- Understanding data relationships: Unstructured data isn’t always standalone. There can be correlations and dependencies between different data sets. Recognizing these relationships can offer valuable insights and shape your data management approach.
2. Data classification and metadata enrichment
As an organization, one of the effective ways to manage your exponentially growing unstructured data is through effective data classification and metadata enrichment. This process involves describing and arranging your data in a manner that makes it easily retrievable and analyzable.
Let’s break down a few helpful strategies for data classification and metadata enrichment:
Formulating a data classification framework
- Understanding the data: Start by creating a clear map of the different types of unstructured data your organization generates or collects. This could include text files, emails, images, audio files, and video content, amongst others.
- Set clear classification criteria: Define the criteria that determine the classification of data. Consider attributes such as the data’s sensitivity level, value to the organization, regulatory requirements, or the level of protection the data requires.
- Designate data stewards: Appoint people or teams who will be responsible for overseeing the data classification process. They need to ensure that all decisions regarding data classification align with the organization’s data policies and business objectives.
- Implement categorization tools: Leverage data classification software or tools. They automate the process of classifying large volumes of unstructured data, thereby saving valuable time and ensuring consistency.
Enhancing data with metadata
Metadata – the data that provides information about other data – can significantly improve how you retrieve and analyze your unstructured data. Here’s how it works:
- Create a metadata schema: This is a framework that outlines what metadata to capture and how to organize it. You’ll want to create a schema that aligns with your data management goals.
- Automate metadata tagging: Use modern technology like AI and machine learning to automate the process of metadata tagging. These technologies can extract keywords, patterns, and meaningful information from large volumes of content, which you can then use to generate metadata tags.
- Data consistency: Ensure metadata is consistent and standardized across all platforms. Consistent metadata allows for easier data retrieval, discovery, and exchange.
- Utilize metadata for data governance: Metadata can support data governance efforts by providing valuable insights into data usage, data quality, and data lineage.
Cleverly planned and executed, data classification and metadata enrichment can significantly simplify the management of unstructured data. They enhance the accessibility and usability of data, helping organizations derive more insights and value from their data assets.
Remember: Unstructured data is not without value—it simply requires the right approaches to unlock its potential. With the right classification and metadata strategy, you’re well on your way there.
3. Implementing robust data governance
Understanding the importance of establishing a robust data governance structure is crucial when dealing with unstructured data. Effective unstructured data management solutions also ensure the maintenance of data quality, essentially increasing your organization’s ability to draw valuable insights from it, enhance security measures, and ensure compliance.
Here are some crucial strategies to guide you:
- Clear data governance policy: Define clear policies and procedures that cover every aspect of data management, including data collection, input, storage, processing, and output. To be effective, these policies should facilitate a strong and structured framework for managing both unstructured and structured data.
- Data stewardship: Assign data stewards to take ownership of data assets. They will ensure data is correctly entered, updated, maintained, and protected from unauthorized access. The stewards can also help ensure that the data generates value for the business.
- Data quality management: Ensure policies around data cleansing and enrichment are adhered to. This can help maintain data reliability, consistency, and accuracy, which will enhance the decision-making process.
- Security and privacy: Implement robust security measures to protect sensitive data and maintain customer faith. Encrypting data, setting up firewalls, and conducting regular security audits are key aspects of this effort. Furthermore, your data governance policies should comply with all relevant privacy laws and regulations to avoid potential legal consequences.
- Data accessibility: Create protocols for data accessibility that outline who can access the different forms of data, when they can access it, and what they can do with it. This is relevant not only for in-house teams but also for third-party vendors or partners.
- Regular monitoring and auditing: Evaluate the effectiveness of your data governance program via regular monitoring and audits. This will help identify any gaps or weaknesses in your process and act as a mechanism for continuous improvement.
4. Leveraging AI and machine learning for data analysis
Unstructured data present within an organization can be a gold mine of insights and valuable information. Harnessing the prowess of Artificial Intelligence (AI) and Machine Learning (ML) can be pivotal to unlocking the hidden potential of this unstructured data.
Here’s how:
- Data pre-processing: Before AI and ML can set to work, they first need to understand the data. Pre-processing involves cleaning and normalizing, transforming textual data into a numerical format with the help of techniques like one-hot encoding and word embeddings. This stage ensures that your data is in a form comprehensible to machine learning models.
- Text mining: Used mainly for text data, text mining helps find latent patterns, significant phrases, words, or sentences within unstructured data. This information can then be used for sentiment analysis, topic modeling, and text classification.
- Natural language processing (NLP): NLP leverages AI to enable machines to understand and interpret human language. Through techniques such as tokenization, lemmatization, and stop-word removal, NLP provides a structured view of the data, which can be instrumental in understanding the sentiment, context, or themes inherent in the data.
- Image and video analytics: AI and ML technologies can efficiently parse through images and video data in search of specified features, patterns, or anomalies. Techniques such as Neural Networks and Convolutional Neural Networks (CNN) are applied for the identification and classification of images, which can greatly aid in enhancing customer experience, security, and efficiency, among others.
- AI-powered search engines: These search engines use ML and AI to provide highly accurate and quick responses to queries by understanding the intent and context behind them. With the help of AI, search operators can function far beyond the capacity of traditional, keyword-based algorithms, providing nuanced insights and relevant answers.
- Sentiment analysis: ML algorithms process vast quantities of unstructured data, such as social media posts, online reviews, and customer feedback, to discern the sentiment behind them. This can be used to gauge customer behavior, satisfaction, feedback, and brand sentiment.
- Automated feature extraction: Feature extraction involves translating raw data into a useful format for predictive modeling. Machine learning automates this process, identifying and creating features from the raw, unstructured data that are most helpful for making accurate predictions.
- Predictive modeling: Advanced ML models are used to predict future outcomes based on the insights derived from unstructured data. From predicting customer churn to forecasting market trends, the possibilities are endless.
The intersection of AI and ML’s unstructured data management tools allows companies to reimagine their approach to unstructured data.
Why leave valuable business insights buried in your unstructured data? Explore the intricacies of leveraging technology to mitigate data risks and elevate data quality, preparing you for the advancements of Gen AI.
5. Continuous data quality improvement
One approach that can significantly improve the management of unstructured data is continuous data quality improvement. Continuous data quality improvement is not simply a one-off task to be ticked off a to-do list; it’s an ongoing journey that keeps pace with evolving data and business needs.
The accuracy, completeness, and reliability of your data are crucial facets that need constant monitoring and fine-tuning.
Confused about where to start? Do not worry; we’ve got you covered. Here are some points you can keep in mind:
- Accuracy: Simply put, inaccurate data is useless. Incorrect information can skew your analytics results and lead to misguided decision-making. Continuous efforts should be made to ensure that data recorded and stored is as accurate as possible and that any inaccuracies detected are corrected promptly.
- Completeness: Missing information can equally distort the picture painted by your data. Regular checks should be made to test the completeness of your data and to identify and fill any gaps in information that might exist.
- Reliability: Additionally, the consistency of your data forms an essential part of its quality. Unreliable data can cast doubt on the insights derived from it, negating the key benefits of data-driven decision-making. As such, measures should be implemented to ensure data reliability through consistency checks and validation processes.
Central to continuous data quality improvement is the understanding that it is an ongoing, iterative process. It is about setting up systems to monitor and update your data constantly, not simply correcting inaccuracies when you stumble upon them.
While the process might be challenging, the rewards in terms of actionable insights, customer satisfaction, and business growth are invaluable.
6. Optimizing unstructured data storage
It’s not easy to store unstructured data; it requires a different perspective as opposed to the traditional data storage methods used for structured data. It cannot be simply inserted into a grid of columns and rows.
Here, we’ll explore several essential elements and effective approaches for storing unstructured data:
- Scalability: As the volume of unstructured data grows, storage solutions must be designed to scale with it. Traditional storage methods may struggle as data volumes increase, making it critical to choose a storage strategy specifically designed for scalability.
- Object storage: Object storage is an ideal solution for storing vast amounts of data. With each piece of data assigned a unique identifier, it offers easy access and fast scalability.
- Cloud-based storage: Cloud solutions offer near-endless scalability and storage management benefits. They ensure seamless information storage and retrieval while offering flexible scalability to match your growing unstructured data volumes.
- Accessibility: Accessibility to data stored, irrespective of its formatted storage, is another crucial aspect of its effective management.
- Enhanced metadata: Enhanced metadata within object storage can help improve accessibility. It’s easier to retrieve and use data when detailed metadata is included. This can also significantly improve searchability for unstructured data.
- Simplified data retrieval: Storage solutions must simplify data retrieval processes to ensure timely and efficient access to unstructured data. Cloud storage and object storage offer benefits in this area, providing easy access to data via the Internet.
- Security: Security measures are paramount given the sensitive nature of some unstructured data. A breach could lead to significant losses for an enterprise.
- Encryption: Implementing robust data encryption, both at rest and in transit, protects unstructured data from unauthorized access.
- Access controls: Setting strict access controls restricts who can access the data. Role-based access, for instance, can be designed to permit only authorized personnel to access sensitive, unstructured data.
- Security compliance: It is vital to ensure that the chosen storage solution complies with current security standards and regulations. Compliance with standards like the General Data Protection Regulation (GDPR) and the Health Insurance Portability and Accountability Act (HIPAA) is particularly crucial when handling sensitive data.
7. Adopting scalable data management solutions
The right scalable data management solution can be the difference between untapped data chaos and structured business growth. Your organization’s future viability lies in adopting flexible, expandable, and adaptable strategies that customize and streamline your handling of unstructured data.
Below, we present several elements vital to these solutions, focusing in particular on storage:
Scalability
Handling unstructured data requires solutions that can grow with your needs without compromising on performance. Systems capable of automatically scaling to manage heightened data load are critical. Unstructured data’s inherent complexity and volume necessitate scalable solutions that can evolve in stride with your business.
Leading cloud providers such as AWS and Microsoft Azure present opportunities for scalable storage that can match your company’s growth pace. Services like these allow you to add storage capacity as required without the need for disruptive, costly infrastructure changes.
Accessibility
Unstructured data’s value isn’t realized until it’s accessed and properly analyzed. Therefore, your selected solution must ensure always-on, secure access to your data. A good data management strategy ensures that the right individuals have access to the necessary data when they need it.
Services like Big Data Development help companies harness the potential of their unstructured data, enabling access to innovative, data-driven decision-making.
Security
In an era of increasing cyber threats, a strong security strategy is non-negotiable. Your unstructured data contains sensitive and valuable information; it must be safeguarded suitably to prevent unauthorized access or breaches.
Robust data governance policies, complemented with the correct security tools, will ensure that your data retains its integrity while still being readily available for authorized use.
Data durability and availability
Your organization needs to ensure that the adopted data management solutions enhance the data’s durability and availability. Data durability ensures that once the data is stored, it remains intact, robust, and uncompromised.
Data availability, on the other hand, ensures that the data is obtainable and usable when necessary. Adopting solutions that uphold these two aspects contributes to maintaining data usability and operational resilience.
By carefully considering these vital factors and investing in a scalable data management solution, your organization can balance data regulation with innovation, liberating the transformative potential of unstructured data.
Streamline your unstructured data management with EncompaaS
Managing unstructured data is critical yet challenging, as it’s a rich source of insights that can enhance decision-making and business outcomes. Plus, dealing with high volumes, diversity, and the accuracy of this data can be daunting.
Here is where EncompaaS comes in. Its powerful AI and machine learning capabilities help classify and analyze unstructured data, turning it into actionable information. EncompaaS is more than a solution; it’s your strategic partner in maximizing the potential of unstructured data with data compliance and privacy.
Explore EncompaaS for improved data management and revolutionize your data strategies today. Book a demo!
FAQs
What are the challenges of managing unstructured data?
The challenges of managing unstructured data include its vast volume and variety, making it difficult to store, process, and analyze efficiently. Unlike structured data, it does not fit neatly into traditional databases, requiring more sophisticated tools and techniques for extraction and interpretation.
Additionally, ensuring data quality and security poses significant hurdles, as the data’s irregular format complicates the consistent application of governance, privacy, and protection standards.
How can businesses effectively categorize and store unstructured data?
Businesses can effectively categorize and store unstructured data by implementing a few key strategies:
- Data Identification and Classification: Machine learning algorithms and natural language processing tools automatically identify and classify data based on content and context.
- Metadata Tagging: Attach metadata tags to unstructured data. These tags include information about the data’s origin, content type, and relevance, which aids in categorization and retrieval.
- Hierarchical Storage Management: Implement storage solutions that organize data into hierarchical tiers. Store frequently accessed data on faster, more expensive storage media and less frequently accessed data on cheaper, slower media.
- Data Lakes: Use data lakes to store vast amounts of raw data in their native format. This allows for greater flexibility in handling various types of unstructured data.
- Cloud Storage Solutions: Leverage cloud storage providers that offer scalability, data durability, and enhanced security features, making them ideal for storing large volumes of unstructured data.
- Advanced Search Capabilities: Integrate advanced search tools and indexing to facilitate the quick retrieval of relevant information from a large pool of unstructured data.
What tools and technologies are best for analyzing unstructured data?
EncompaaS is a valuable tool for managing and analyzing unstructured data. It provides governance automation, helping businesses ensure compliance across various data types and sources. EncompaaS integrates seamlessly with existing systems to enhance data visibility and control, making it easier to apply compliance rules and analyze unstructured data for actionable insights.
How does managing unstructured data improve business decision-making?
Managing unstructured data improves business decision-making by providing deeper insights from diverse data sources like emails, social media, and videos. It allows organizations to uncover hidden patterns, customer sentiments, and market trends. This enriched data pool supports more informed, data-driven decisions, enhances customer engagement strategies, and drives innovative solutions by leveraging information previously untapped due to its complex nature.
Book a demo
Let's get started
Experience the Power of EncompaaS!
Submit this form to see EncompaaS in action with a demo from our information management experts.